MRI Plus Deep Learning Models Estimate Brain-Aging
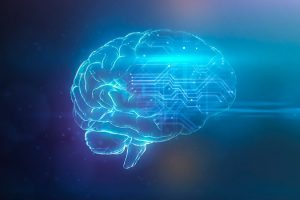
The study covered in this summary was published in bioRxiv.org as a preprint and has not yet been peer reviewed.
Key Takeaways
-
Using the Layer-wise Relevance Propagation algorithm and various structural MRI modalities (T1-weighted, fluid-attenuated inversion recovery [FLAIR], and susceptibility-weighted magnitude images [SWI]), brain age can be estimated.
-
The structural abnormalities found were changes in the gray matter and white matter, such as atrophy in the cortex, subcortex, cerebellum, and brainstem, in addition to global brain shrinkage (shown by the larger size of ventricles and sulci).
Why This Matters
-
The Layer-wise Relevance Propagation algorithm, a deep learning model, is a bias-free computational method of estimating brain-aging that can help look at brain-aging globally and in terms of pathomechanisms. This can help detect brain-aging in healthy individuals and individuals at higher risk during adulthood and potentially be used in personalizing medicine.
Study Design
-
MRI data from participants aged 18-82 years from the LIFE adult study (a population-based cohort study) were included in this study. Patients with various prior neurologic disorders were excluded.
-
The participants had undergone a 1-hour MRI (T1-weighted, FLAIR, and SWI).
-
The MRIs were used to make models, and then multilevel ensembles were made of the models. The areas of the brain that were looked at with these sub-ensembles were the cerebellum, subcortical, and cortical areas.
-
The Layer-wise Relevance Propagation algorithm was then used to predict brain- age.
-
An exploratory analysis was also done to correlate diverging brain-age with certain risk factors that are known to be associated with accelerated brain age (cardiometabolic, genetic, and neural integrity).
Key Results
-
Qualitative Layer-wise Relevance Propagation analysis showed brain areas that had higher or lower brain-age predictions. The areas in and around the ventricles and at the border from the brain to meningeal areas had large contributions, independent of MRI sequence; most of the white-matter areas were not as helpful, except those white matter lesions in the FLAIR images.
-
In the older participants, the areas covering cortical sulcal structures were more contributory than they were in younger participants and showed an older brain-age.
-
In both younger and older participants, the corpus callosum, the brain stem and areas in and around the cerebellum contributed information. The gray matter, white matter, and cortical spinal fluids showed a linear increase of relevance scores as function of age; this was strongest in the gray matter, and weakest in the spinal fluid.
-
The brain structures (areas around the ventricles and the sulci in the cortex and cerebellum) were found to be important for the multilevel ensembles’ predictions.
-
The base models using the T1 sub-ensemble had the most information in the lateral ventricle, corpus callosum, pre- and postcentral gyri in the motor and sensorimotor cortex, operculum, and all gray matter border areas. The FLAIR sub-ensemble had the most information around the lateral ventricles, anterior temporal gyri, the pre- and postcentral gyri, and white matter areas. The base models of the SWI sub-ensemble had a stronger focus on the gray matter areas in the visual pole and occipital lobe, limbic areas, corpus callosum, white matter fornix, internal capsule and on subcortical nuclei and brainstem areas.
-
A comparison of the Layer-wise Relevance Propagation heatmaps of the older cohort vs the younger cohort showed that there was greater relevance in the older brains compared to younger brains (P = .5) in the T1 sub-ensemble in the lateral ventricles, corpus callosum, amygdala, cerebral white matter (especially in the paracingulate gyrus), opercular cortex, and (secondary) somatosensory cortex. For the FLAIR sub-ensemble, the increase was in the cerebellum (specifically, left and right crus I-II), caudate, inferior frontal gyrus, pars triangularis, insular cortex, and inferior parietal lobule. For the SWI sub-ensemble, the relevance was higher in the frontal pole, frontal orbital cortex, inferior frontal gyrus, pars triangularis, precuneus, basal nuclei, and occipital pole.
-
The Layer-wise Relevance Propagation relevance maps were also used to look at the role of health-related risk factors on brain-age. Patients with type 2 diabetes were compared with healthy subjects, aged 50-75 years. In the T1 sub-ensemble, there was higher positive relevance (non-healthy > healthy) that was significant in the pre- and postcentral gyrus near the cortico-spinal tract in the primary motor cortex (P = .011), corpus callosum and cingulum (P = .02. For the FLAIR and SWI sub-ensembles there were no significant regional differences.
-
Diverging brain-age (accelerated aging) was found to have higher relevance in the different sub-ensembles (P = .05 for all). For the T1 sub-ensembles, the difference was found in the frontal pole, brain stem, outer cerebellar borders, the white matter (including the cortical spinal tract), putamen, caudate, amygdala, pre- and post-central gyri, and cingulate gyri. For the FLAIR sub-ensemble, the associations were found in the occipital and parietal pole, lingual gyrus, and cerebellum. For the SWI sub-ensembles, posterior and anterior regions showed significant associations, including the frontal pole, frontal orbital cortex, occipital pole, cerebellum, and left-lateral parieto-temporal white matter structures close to the putamen and operculum.
-
In the younger cohort (age < 45 years), higher diverging brain-age correlated with cardiovascular risk factors (hypertension and hyperlipidemia). In the older subjects (older than age 60) the most prevalent positive association of diverging brain-age was with type 2 diabetes, with a weaker association with glycated hemoglobin levels. In all ages, body mass index, waist-to-hip ratio, and white matter lesion load had positive associations with diverging brain-age.
Limitations
-
The Layer-wise Relevance Propagation heatmaps do not explain exactly what the biologic mechanisms are that make specific areas relevant.
-
Using aggregation techniques for the relevance maps might have caused loss of information.
-
The ages of the subjects are mostly between 65-75 years, which introduces bias.
Disclosures
-
The study was supported by the European Union, European Regional Development Fund as part of the LIFE-LIFT project, the Free State of Saxony, the LIFE-Leipzig Research Center for Civilization Diseases, University of Leipzig, the German Research Foundation, the German Ministry for Education and Research (BMBF) through Berlin Institute for the Foundations of Learning and Data, Transparent Medical Expert Companion, European Union’s Horizon 2020 research and innovation program through Intelligent Total Body Scanner for Early Detection of Melanoma, Deutsche Forschungsgemeinschaft (DFG), and the Institute of Information & Communications Technology Planning & Evaluation (IITP) grant funded by the Korea Government.
-
The authors have no competing interests.
This is a summary of a preprint research study, “Towards the Interpretability of Deep Learning Models for Multi-modal Neuroimaging: Finding Structural Changes of the Aging Brain,” written by Simon M. Hofmann, MSc, from the Department of Neurology, Max Planck Institute for Human Cognitive and Brain Sciences, the Clinic for Cognitive Neurology, University of Leipzig Medical Center, Leipzig, and the Department of Artificial Intelligence, Fraunhofer Institute Heinrich Hertz, Berlin, Germany, and colleagues, on bioRxiv, provided to you by Medscape. This study has not yet been peer reviewed. The full text of the study can be found on bioRxiv.org.
For more news, follow Medscape on Facebook, Twitter, Instagram, YouTube, and LinkedIn
Source: Read Full Article