New method to enhance images of the SARS-CoV-2 virus

Researchers report a method of removing noise from high-resolution transmission electron microscopy images using probabilistic generative models.
Electron microscopy (EM) is a technique used to see viruses and their infection processes, allowing us to see how it binds to cells and spreads through the body. Transmission electron microscopy (TEM) can provide high-resolution images at the nanometer scale, but these low-light images generally have an abundance of noise. Images of viruses often appear flat or 2-dimensional.
Several noise reduction algorithms have helped improve image quality, allowing better interpretation of the data. For the severe acute respiratory syndrome coronavirus 2 (SARS-CoV-2), EM images have also been used to reconstruct 3-dimensional (3D) images of the virus.
This generally involves using a large number of TEM images, for example, to generate 3D structures of proteins. A study used cryoelectron tomography to record many TEM images of the virus as the sample is tilted along an axis, and the obtained images are merged using software. If only noisy images are available, typical approaches such as feed-forward neural networks cannot be used as they need a large number of pairs of clean and noisy images.
Denoising a single TEM image
In a new study published on the bioRxiv* preprint server, researchers from Carl von Ossietzky University Oldenburg report a method to obtain 3D images of the virus using a single TEM image.
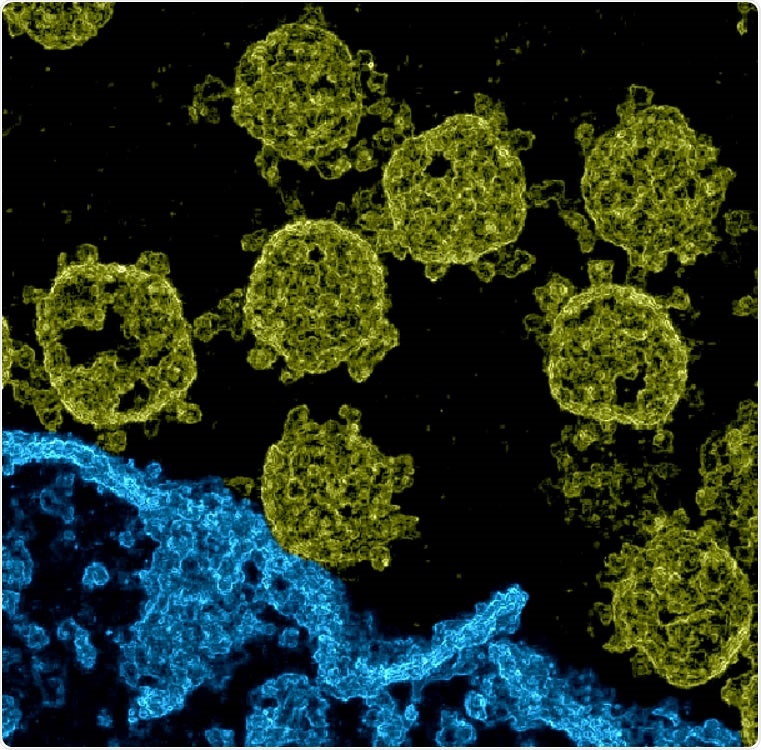
They used probabilistic generative models first to learn data representations of the TEM images. These approaches are unsupervised and generally need fewer data to learn the suitable representations. If only one image is available for learning, image processing is complicated. However, intrinsic image statistics often have high-quality information, which can be used.
The researchers used a freely available TEM image of SARS-CoV-2 in Vero cell cultures and used noisy data in the form of patches extracted from the image. After cropping the image to a smaller size, they halved the pixel density by removing every second pixel to reduce computational demand. Their approach to generating the denoised image was by estimating the mean of each pixel.
They also processed the same image using other probabilistic models. Using a Gamma-Poisson mixture model changes the spatial appearance and to a lesser extent the appearance of the conventionally denoised image. The models that give explicit probabilistic distribution for image patches are important, whereas non-local image processing methods were less suited.
Visual inspection of the denoised image proved the quality was similar to those obtained using state-of-the-art algorithms and effective noise suppression in high-resolution EM images.
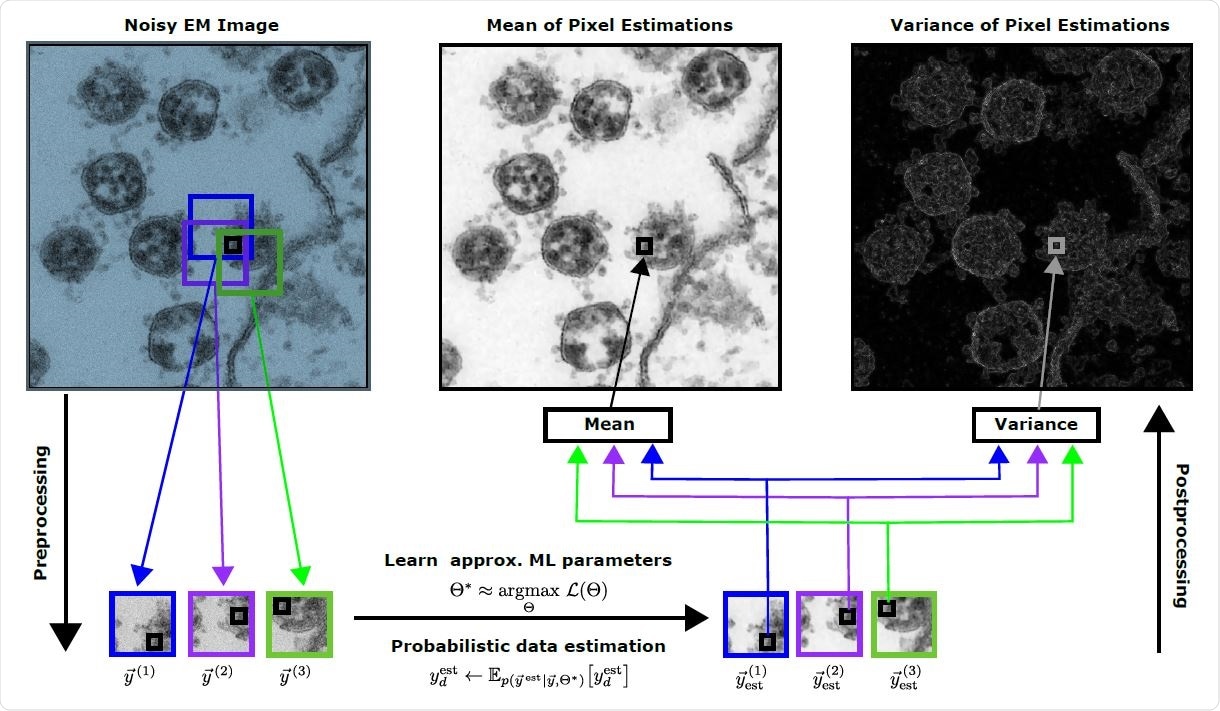
Advantages and disadvantages
The team also analyzed the reconstruction statistics. The pixel variance estimations can also be used to provide a spatial impression of the infection area. Although previous tomography image reconstruction can provide high-quality images, they require many TEM images of a single virus, which is difficult to obtain. This method cannot reconstruct 3D images of an entire infection scene.
Each method used for 3D representation can introduce its own artifacts. For example, artifacts can be present in the new method because there is less representation of rare structures. In addition, since the reconstruction is based on a single image, actual surfaces are not estimated.
An advantage of the new method is that surfaces do no obstruct other structures, but a disadvantage is that internal virus structures can become entangled with surface structures. Since different methods have different advantages, they can be combined into hybrid systems.
Different approaches are generally used for different data. For example, the protein reconstruction method reported before combines TEM images of several protein molecules of different viruses, which the new method cannot do. The new approach cannot combine many TEM images of the same virus to generate a 3D image. But, the other methods cannot recover a spatial impression from a single noisy image.
Although spatial images of virus infections have been reported before using scanning electron microscopy images, they have a low resolution and do not show the details of the virus. The images obtained using the new method have a high level of detail for the SARS-CoV-2, which may better understand the infection.
The colored and the original image are available for download at this repository.
*Important Notice
bioRxiv publishes preliminary scientific reports that are not peer-reviewed and, therefore, should not be regarded as conclusive, guide clinical practice/health-related behavior, or treated as established information.
- Drefs, J. et al. (2021) Visualization of SARS-CoV-2 Infection Scenes by 'Zero-Shot' Enhancements of Electron Microscopy Images. bioRxiv. https://doi.org/10.1101/2021.02.25.432265, https://www.biorxiv.org/content/10.1101/2021.02.25.432265v1
- Drefs, Jakob, Salwig, Sebastian, & Lücke, Jörg. (2021, February 25). Visualization of SARS-CoV-2 Infection Scenes by "Zero-Shot" Enhancements of Electron Microscopy Images. Zenodo. http://doi.org/10.5281/zenodo.4559517
Posted in: Device / Technology News | Medical Research News | Disease/Infection News
Tags: Cell, Coronavirus, Coronavirus Disease COVID-19, Electron, Electron Microscopy, Machine Learning, Microscopy, Protein, Respiratory, SARS, SARS-CoV-2, Severe Acute Respiratory, Severe Acute Respiratory Syndrome, Syndrome, Tomography, Virus
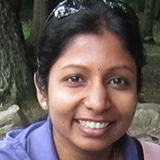
Written by
Lakshmi Supriya
Lakshmi Supriya got her BSc in Industrial Chemistry from IIT Kharagpur (India) and a Ph.D. in Polymer Science and Engineering from Virginia Tech (USA).
Source: Read Full Article